A simulation study on the insurance claims distribution using Weibull distribution
DOI:
https://doi.org/10.58567/eal02030008Keywords:
Weibull distribution; Bayesian Estimator; Maximum Likelihood Estimator; Insurance ClaimAbstract
The Weibull distribution is extensively useful in the field of finance, insurance and natural disasters. Recently, It has been considered as one of the most frequently used statistical distributions in modelling and analyzing stock pricing movement and uncertain prediction in financial and investment data sets, such as insurance claims distribution. It is well known that the Bayes estimators of the two-parameter Weibull distribution do not have a compact form and the closed-form expression of the Bayes estimators cannot be obtained. In this paper and the Bayesian setting, it is assumed that the scale parameter of the Weibull model has a gamma prior under the assumption that its shape parameter is known. A simulation study is performed using random claims amount to compare the performance of the Bayesian approach with traditional maximum likelihood estimators in terms of Root Mean Square Errors (RMSE) and Mean Absolute Error (MAE) for different sample sizes, with specific values of the scale parameter and shape parameters. The results have been compared with the estimated result via the maximum likelihood method. The result revealed that the Bayesian approach behaves similarly to the maximum likelihood method when the sample size is small. Nevertheless, in all cases for both methods, the RMSE and MAE decrease as the sample size increases. Finally, applications of the proposed model to the insurance claim data set have been presented.
References
Abubakar, H., and Muhammad Sabri, S. R. (2021). A Simulation Study on Modified Weibull Distribution for Modelling of Investment Return. Pertanika Journal of Science and Technology, 29(4). https://doi.org/10.47836/pjst.29.4.29
Abubakar, H., and Sabri, S. R. M. (2022). Simulated Annealing Algorithm as Heuristic Search Method in the Weibull Distribution for Investment Return Modelling. In Digital Economy, Business Analytics, and Big Data Analytics Applications (pp. 401–414). Springer. https://link.springer.com/chapter/10.1007/978-3-031-05258-3_32
Abubakar, H., and Sabri, S. R. M. (2023). A Bayesian Approach to Weibull Distribution with Application to Insurance Claims Data. Journal of Reliability and Statistical Studies, 1–24. https://doi.org/10.13052/jrss0974-8024.1611
Ahmad, Z., Mahmoudi, E., and Hamedani, G. (2020). A class of claim distributions: Properties, characterizations and applications to insurance claim data. Communications in Statistics - Theory and Methods. https://doi.org/10.1080/03610926.2020.1772306
Almetwally, E. M., and Almongy, H. M. (2021). Maximum Product Spacing and Bayesian Method for Parameter Estimation for Generalized Power Weibull Distribution Under Censoring Scheme. Journal of Data Science. https://doi.org/10.6339/jds.201904_17(2).0010
Charles, V., Tsolas, I. E., and Gherman, T. (2018). Satisficing data envelopment analysis: A Bayesian approach for peer mining in the banking sector. Annals of Operations Research. https://doi.org/10.1007/s10479-017-2552-x
Chen, W., Shirzadi, A., Shahabi, H., Ahmad, B. Bin, Zhang, S., Hong, H., and Zhang, N. (2017). A novel hybrid artificial intelligence approach based on the rotation forest ensemble and naïve Bayes tree classifiers for a landslide susceptibility assessment in Langao County, China. Geomatics, Natural Hazards and Risk. https://doi.org/10.1080/19475705.2017.1401560
Ching, J., and Phoon, K.-K. (2019). Constructing Site-Specific Multivariate Probability Distribution Model Using Bayesian Machine Learning. Journal of Engineering Mechanics. https://doi.org/10.1061/(asce)em.1943-7889.0001537
Henclová, A. (2006). Notes on free lunch in the limit and pricing by conjugate duality theory. Kybernetika. https://doi.org/10.18452/2963
Hersch, G. (2019). No Theory-Free Lunches in Well-Being Policy. Review of Financial Studies. https://doi.org/10.1093/pq/pqz029
Kaminskiy, M. P., and Krivtsov, V. V. (2005). A simple procedure for Bayesian estimation of the Weibull distribution. IEEE Transactions on Reliability. https://doi.org/10.1109/TR.2005.858093
Köksal Babacan, E., and Kaya, S. (2019). A simulation study of the Bayes estimator for parameters in Weibull distribution. Communications Faculty Of Science University of Ankara Series A1Mathematics and Statistics. https://doi.org/10.31801/cfsuasmas.455276
Kowal, D. R., Matteson, D. S., and Ruppert, D. (2017). A Bayesian Multivariate Functional Dynamic Linear Model. Journal of the American Statistical Association. https://doi.org/10.1080/01621459.2016.1165104
Nassar, M., Afify, A. Z., Dey, S., and Kumar, D. (2018). A new extension of Weibull distribution: Properties and different methods of estimation. Journal of Computational and Applied Mathematics. https://doi.org/10.1016/j.cam.2017.12.001
Park, S., Choi, S., and Jun, S. (2021). Bayesian structure learning and visualization for technology analysis. Sustainability (Switzerland). https://doi.org/10.3390/su13147917
Shakhatreh, M. K., Dey, S., and Alodat, M. T. (2021). Objective Bayesian analysis for the differential entropy of the Weibull distribution. Applied Mathematical Modelling. https://doi.org/10.1016/j.apm.2020.07.016
Sultan, K. S., Alsadat, N. H., and Kundu, D. (2014). Bayesian and maximum likelihood estimations of the inverse Weibull parameters under progressive type-II censoring. Journal of Statistical Computation and Simulation. https://doi.org/10.1080/00949655.2013.788652
Tung, Y. L., Ahmad, Z., Kharazmi, O., Ampadu, C. B., Hafez, E. H., and Mubarak, S. A. M. (2021). On a New Modification of the Weibull Model with Classical and Bayesian Analysis. Complexity. https://doi.org/10.1155/2021/5574112
Vasishth, S., Nicenboim, B., Beckman, M. E., Li, F., and Kong, E. J. (2018). Bayesian data analysis in the phonetic sciences: A tutorial introduction. Journal of Phonetics. https://doi.org/10.1016/j.wocn.2018.07.008
Yanuar, F., Yozza, H., and Rescha, R. V. (2019). Comparison of Two Priors in Bayesian Estimation for Parameter of Weibull Distribution. Science and Technology Indonesia. https://doi.org/10.26554/sti.2019.4.3.82-87
Zaidi, A., Bouamama, B. O., and Tagina, M. (2012). Bayesian reliability models of weibull systems: State of the art. International Journal of Applied Mathematics and Computer Science. https://doi.org/10.2478/v10006-012-0045-2
Zhang, Y. (2017). Bayesian analysis of big data in insurance predictive modeling using distributed computing. ASTIN Bulletin. https://doi.org/10.1017/asb.2017.15
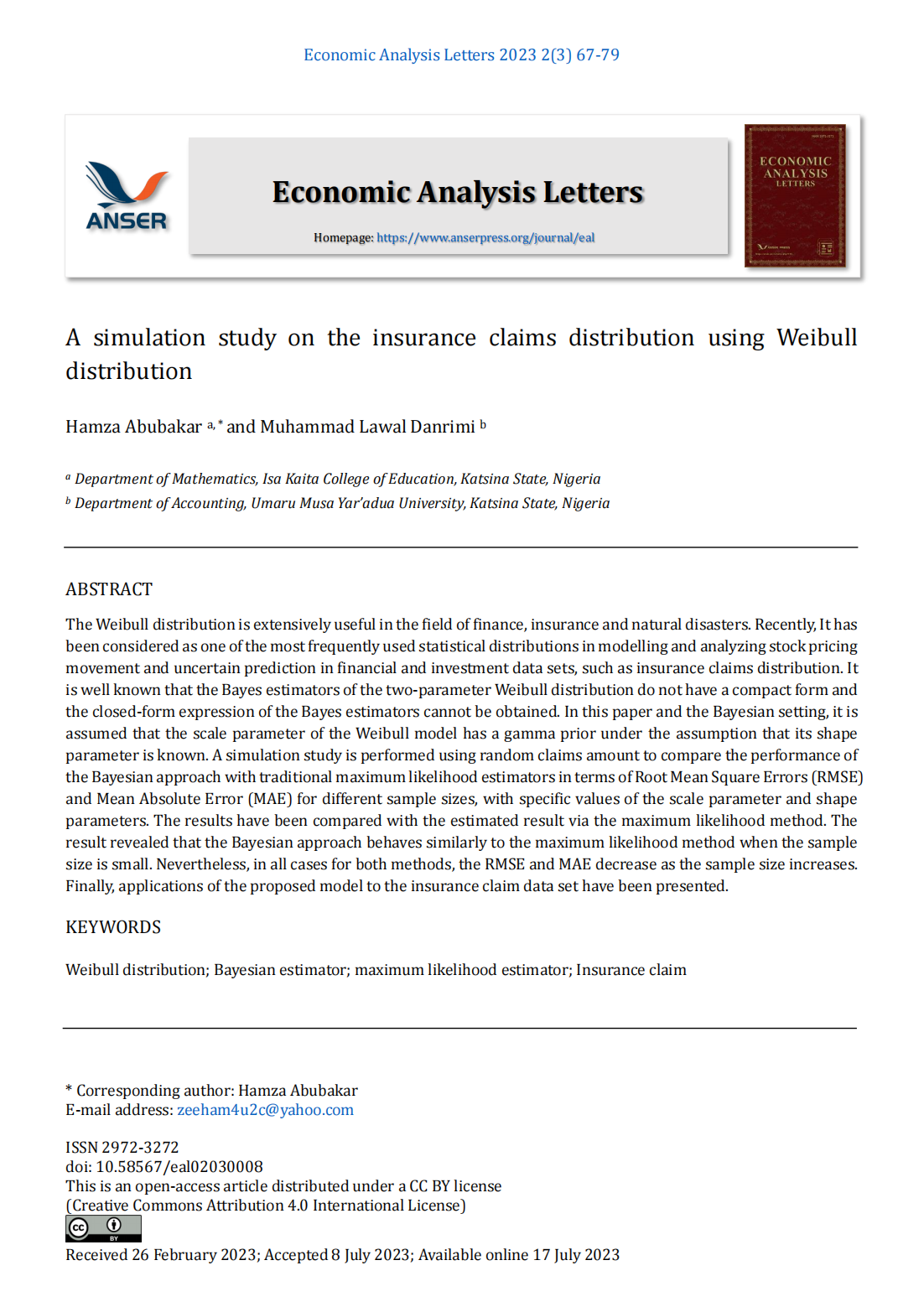
Downloads
Published
How to Cite
Issue
Section
License
Copyright (c) 2023 Hamza Abubakar, Muhammad Lawal Danrimi

This work is licensed under a Creative Commons Attribution 4.0 International License.