Manufacturing Cost Estimation Using Piecewise Function Approaches
DOI:
https://doi.org/10.58567/jea02030007Keywords:
Credit scoring; artificial intelligence; discriminant analysis; logistic regression; artificial neural networks; random forestAbstract
This paper describes two novel approaches to cost estimation of manufactured products where a data set of similar products have known manufactured costs. The methods use the notion of piecewise functions and are (1) clustering and (2) splines. Cost drivers are typically a mixture of categorical and numeric data which complicates cost estimation. Both clustering and splines approaches can accommodate this. Through four case studies, we compare our approaches with the often-used regression models. Our results show that clustering especially offers promise in improving the accuracy of cost estimation. While clustering and splines are slightly more complex to develop from both a user and a computational perspective, our approaches are packaged in an open-source software. This paper is the first known to adapt and apply these two well-known mathematical approaches to manufacturing cost estimation.
References
Al-Sultan, K. S. (1995). A tabu search approach to the clustering problem. Pattern Recognition 28(9), 1443-1451. https://doi.org/10.1016/0031-3203(95)00022-R
Almond, D., Chay, K. Y., & Lee, D. S. (2005). The cost of low birth weight. The Quarterly Journal of Economics 120(3), 1031-1084. https://doi.org/10.1093/qje/120.3.1031
Angelis, L. & Stamelos, I. (2000). A simulation tool for efficient analogy based cost estimation. Empirical Software Engineering 5(1), 35-68. https://doi.org/10.1023/A:1009897800559
Audet, C., Le Digabel, S. & Tribes, C. (2009). NOMAD user guide. Les cahiers du GERAD, Technical Report G-2009-37. https://www.gerad.ca/fr/papers/G-2009-37.pdf
Baker, F. B. & Hubert, L. J. (1975). Measuring the power of hierarchical cluster analysis. Journal of the American Statistical Association 70(349), 31-38. https://doi.org/10.2307/2285371
Carides, G. W., Heyse, J. F. & Iglewicz, B. (2000). A regression-based method for estimating mean treatment cost in presence of right-censoring. Biostatistics 1(3), 299-313. https://doi.org/10.1093/biostatistics/1.3.299
Chang, W. (2016). Package 'shiny': Web application framework for R, R Package version 0.13.2. Retrieved from https://github.com/rstudio/shiny/
Cheng, C.-H. (1995). A branch and bound clustering algorithm. IEEE Transactions on Systems, Man and Cybernetics 25(5), 895-898.
Curry, H. B. & Schoenberg, I. J. (1947). On spline distributions and their limits: The Polya distribution functions. Bulletin of the American Mathematical Society 53, no. 1114.
Dai, J. S., Niazi, A., Balabani, S. & Seneviratne, L. (2006). Product cost estimation: Technique classification and methodology review. Journal of Manufacturing Science and Engineering 128(2), 563-575. https://doi.org/10.1115/1.2137750
Dalrymple-Alford, E. C. (1970). Measurement of clustering in free recall. Psychological Bulletin 74(1), 32-34.
de Boor, C. (1976). A Practical Guide to Splines. New York: Springer-Verlag.
Dunn, J. C. (1973). A fuzzy relative of the ISODATA process and its use in detecting compact well-separated clusters. Journal of Cybernetics 3(3), 32-57.
Eilers, P. H. C. & Marx, B. D. (1996). Flexible smoothing with B-splines and penalties. Statistical Science 11(2), 89-102. https://doi.org/10.1214/ss/1038425655
Everitt, B. S., Landau, S., Leese, M., & Stahl, D. (2010). Cluster Analysis. Chichester: John Wiley & Sons.
Goodman, L. A., & Kruskal, W. H. (1954). Measures of association for cross classifications. Journal of the American Statistical Association 49(268), 732-764.
Gower, J. C. (1971). A general coefficient of similarity and some of its properties. Biometrics 27(4), 857-871.
Huang, Z. (1997). Clustering large data sets with mixed numeric and categorical values. Proceedings of the 1st Pacific-Asia Conference on Knowledge Discovery and Data Mining Conference, 21-34.
Huang, Z. (1998). Extensions to the k-means algorithm for clustering large data sets with categorical values. Data Mining and Knowledge Discovery 2(3), 283-304.
Jones, D. R., & Beltramo, M. A. (1991). Solving partitioning problems with genetic algorithms. Proceedings of the Fourth International Conference on Genetic Algorithms, 442-449.
Kaufmann, L., & Rousseeuw, P. (1987). Clustering by means of medoids. In Statistical Data Analysis Based on the L1-norm and Related Methods, 405-416. Amsterdam: Springer.
Kaufmann, L., & Rousseeuw, P. J. (1990). Finding Groups in Data. New York: John Wiley & Sons.
Koontz, W. L. G., Narendra, P. M., & Fukunaga, K. (1975). A branch and bound clustering algorithm. IEEE Transactions on Computers 24(9), 908-915.
Layer, A., Brinke, E.T., Houten, F.V., Kals, H., & Haasis, S. (2002). Recent and future trends in cost estimation. International Journal of Computer Integrated Manufacturing, 15(6), 499-510. https://doi.org/10.1080/09511920210143372
Lee, A., Cheng, C.H., & Balakrishnan, J. (1998). Software development cost estimation: integrating neural network with cluster analysis. Information & Management, 34(1), 1-9. https://doi.org/10.1016/S0378-7206(98)00041-X
Li, Q., & Racine, J.S. (2007). Nonparametric Econometrics: Theory and Practice. Princeton University Press.
Ma, S., Racine, J.S., & Yang, L. (2014). Spline regression in the presence of categorical design predictors. Journal of Applied Econometrics, 10(5), 705-717. https://www.jstor.org/stable/26609055
MacQueen, J. (1967). Some methods for classification and analysis of multivariate observations. Proceedings of Fifth Berkeley Symposium on Mathematical Statistics and Probability, 1, 281-297.
Michaud, K., Messer, J., Choi, H.K., & Wolfe, F. (2003). Direct medical costs and their predictors in patients with rheumatoid arthritis. Arthritis and Rheumatism, 48(10), 2750-2762. https://doi.org/10.1002/art.11439
Milligan, G.W., & Cooper, M.C. (1985). An examination of procedures for determining the number of clusters in a data set. Psychometrika, 50(2), 159-179.
Monmarché, N., Slimane, M., & Venturini, G. (1999). On improving clustering in numerical databases with artificial ants. In Advances in Artificial Life (pp. 626-635). Springer. https://link.springer.com/chapter/10.1007/3-540-48304-7_83
Nie, Z., & Racine, J.S. (2012). The crs package: Nonparametric regression splines for continuous and categorical predictors. The R Journal, 4.2, 48-56. https://doi.org/10.32614/RJ-2012-012
Omran, M., Salman, A., & Engelbrecht, A.P. (2002). Image classification using particle swarm optimization. Proceedings of the 4th Asia-Pacific Conference on Simulated Evolution and Learning, 370-374. https://link.springer.com/chapter/10.1007/978-3-540-34956-3_6
Pahariya, J.S., Ravi, V., & Carr, M. (2009). Software cost estimation using computational intelligence techniques. World Congress on Nature and Biologically Inspired Computing, 849-854 https://doi.org/10.1109/NABIC.2009.5393534 .
Pal, N.R., Bezdek, J.C., & Tsao, E.C.-K. (1993). Generalized clustering networks and Kohonen's self-organizing scheme. IEEE Transactions on Neural Networks, 4(4), 549-557. https://doi.org/10.1109/72.238310
Racine, J.S., Nie, Z., & Ripley, B.D. (2014). Package 'crs': Categorical regression splines. R Package version 0.15-24. Retrieved from https://github.com/JeffreyRacine/R-Package-crs/
Rousseeuw, P.J. (1987). Silhouettes: A graphical aid to the interpretation and validation of cluster analysis. Journal of Computational and Applied Mathematics, 20, 53-65.
SAS/STAT 9.2 User's Guide. (2008). SAS Institute Inc.
Schumaker, L.L. (2007). Spline Functions: Basic Theory. Cambridge University Press. https://doi.org/10.1017/CBO9780511618994
Selim, S.Z., & Al-Sultan, K.S. (1991). A simulated annealing algorithm for the clustering problem. Pattern Recognition, 24(10), 1003-1008. https://doi.org/10.1016/0031-3203(95)00022-R
Sneath, P.H.A. (1957). The application of computers to taxonomy. Microbiology, 17(1), 201-226.
Sokal, R.R., & Michener, C.D. (1958). A statistical method for evaluating systematic relationships. University of Kansas Scientific Bulletin, 38, 1409-1438.
Sørenson, T. (1948). A method of establishing groups of equal amplitude in plant sociology based on similarity of species and its application to analyses of the vegetation on Danish commons. Biologiske Skrifter, 5, 1-34.
Valverde, S.C., & Humphrey, D.B. (2004). Predicted and actual costs from individual bank mergers. Journal of Economics and Business, 56, 137-157. https://doi.org/10.1016/j.jeconbus.2003.05.001
Van Hai, V., Nhung, H.L.T.K., Prokopova, Z., Silhavy, R., & Silhavy, P. (2022). Toward improving the efficiency of software development effort estimation via clustering analysis. IEEE Access, 10, 83249-83264. https://doi.org/10.1109/ACCESS.2022.3185393 .
Ward Jr, J.H. (1996). Hierarchical grouping to optimize an objective function. Journal of the American Statistical Association, 58(301), 236-244.
Wolfe, J.H. (1970). Pattern clustering by multivariate mixture analysis. Multivariate Behavioral Research, 5(3), 329-350.
Xu, Z., & Khoshgoftaar, T.M. (2004). Identification of fuzzy models of software cost estimation. Fuzzy Sets and Systems, 145(1), 141-163. https://doi.org/10.1016/j.fss.2003.10.008
Zahn, C.T. (1971). Graph-theoretical methods for detecting and describing gestalt clusters. IEEE Transactions on Computers, 100(1), 68-86. doi: 10.1109/T-C.1971.223083
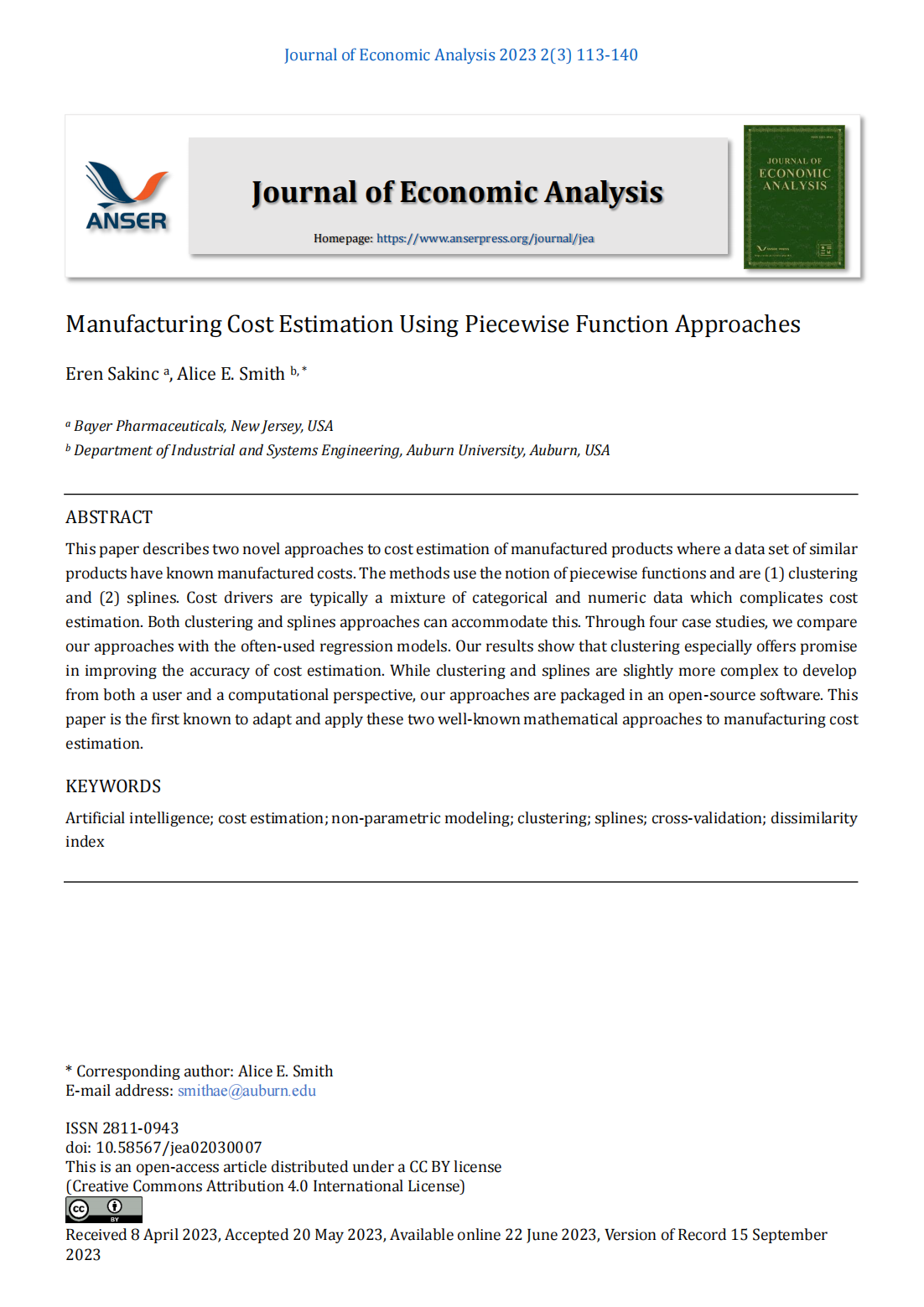
Downloads
Published
How to Cite
Issue
Section
License
Copyright (c) 2023 Eren Sakinç, Alice Smith

This work is licensed under a Creative Commons Attribution 4.0 International License.